7 An Exploratory Study of What Different Theories Can Tell Us about Change
Mary E. Pilgrim, Kelly K. McDonald, Erika G. Offerdahl, Katherine Ryker, Susan Shadle, Amelia Stone-Johnstone, and Emily M. Walter
1 Introduction
National efforts to transform undergraduate STEM education are numerous and diverse, striving to effect change by working with individual faculty, departments, and institutions. Because change is so complex, it can be difficult to determine which theories to use and what affordances specific theories provide. However, without a firm connection to theory, communicating and extending lessons learned from one change project to another is challenging. Presently, there is a need for STEM scholars to become more adept at applying theory to inform STEM transformation initiatives and scholarship.
Institutions are complex systems and instigating change within them is seldom a linear process (Austin, 2011). Organizational change experts argue that catalyzing and sustaining institutional change requires a systems approach (e.g., Henderson et al., 2011; Kezar, 2011). This method explicitly acknowledges that the desired change (e.g., reformed instruction) requires shifts in individual faculty behavior (e.g., instructional practice), and that individuals are embedded in and influenced by a complex institutional context (e.g., Austin, 2011; Kezar, 2011; Reinholz & Apkarian, 2018). From a practical perspective, undergraduate STEM transformation efforts tend to target one or two levels. For example, Departmental Action Teams (Reinholz et al., 2019) and summer institutes (Howard Hughes Medical Institute, 2019) target only departmental and individual levels, respectively. This is out of necessity, as it is often impractical for change agents and scholars to implement and study change across an entire system. Understanding the efficacy of an effort, how to improve it, and how to apply the successes of one project to another requires mutual understanding of how efforts at one level (e.g., individual, department) function within the larger system.
We adopted an Appreciative Inquiry approach (Cooperrider & Srivastva, 1987) to gain clarity about how theory operates to help one understand change generally and how theory can help one understand change from a systems perspective. Appreciative Inquiry is an approach that seeks to affirm and build on existing strengths, potentials, and successes to generate practical and sociorational (interpretive) knowledge about a complex system. Within the context of the exploration described here, the Appreciative Inquiry approach was appropriate because it helped make explicit the various affordances and insights revealed by applying multiple lenses to an existing dataset. It also generated practical implications from grounded observations and collaborative dialogue. We describe here how a team of STEM researchers, discipline-based educational research scholars, and change practitioners applied three commonly used change theories that operate at different scales (e.g., individual, department, organization) to examine a single set of data from an institutional change project. This work serves as a rich case study in the application of theory to STEM education change efforts.
2 Methods
2.1 Data
We used data that had been collected at the start of a department-level change project for undergraduate STEM departments. The change effort was informed by the Change, Adopters, Change Agent, Organization (CACAO) model (Dormant, 2011). During department meetings, faculty were introduced to a vision statement outlining a desired future state for STEM teaching consistent with the use of evidence-based instructional practices (EBIPs). Faculty wrote down ideas about what might support movement toward the vision (drivers) and what might get in the way of achieving the vision (barriers). In the original project, this barrier and driver data was collected and used to understand the context for change in each department (Shadle et al., 2017). For the inquiry reported in this study, a subset of the full data set was used; barrier and driver data from two STEM departments with different contexts (having different barrier-driver profiles) were examined.
2.2 Selection of Theories
We reviewed a variety of theories both familiar and unfamiliar to our research team in order to understand the purpose and function of different change theories. From this list, we selected three that operate across different organizational scales: the Four-Frame model (e.g., Bolman & Deal, 2008; Reinholz & Apkarian, 2018); Rogers’ (2003) Innovation-Decision model; and Ajzen’s (1985) Theory of Planned Behavior (TPB). The Four-Frame model operates at a department/unit, institutional, or organizational level and recognizes that each is comprised of individuals. The Innovation-Decision model examines stages through which individuals make decisions to adopt instructional innovations as influenced by information that comes to/from others or the institution (Rogers, 2003). Lastly, the TPB focuses on individual behaviors and behavioral intent within an institutional context, which are determined by the person’s attitudes, perceived norms, and perceived behavioral controls (Ajzen, 1985).
2.3 Appreciative Inquiry Approach
We approached this process through the lens of Appreciative Inquiry, “an ongoing co-construction of reality” (Cooperrider & Srivastva, 2017, p. 6) that melds theory and practice in action research. In their foundational work, Cooperrider and Srivastva (1987) pose the question, “To what extent does this theory present provocative new possibilities for social action, and to what extent does it stimulate cooperative and normative dialogue about how we can and should organize ourselves?” (p. 98). We responded to this call by posing the following questions:
- What new insights does each theory reveal about the change project?
- In what ways does each theory constrain interpretations of the data?
- How does each theory lead to identification of additional data collection and analyses?
- What next steps does each theory suggest for change agents?
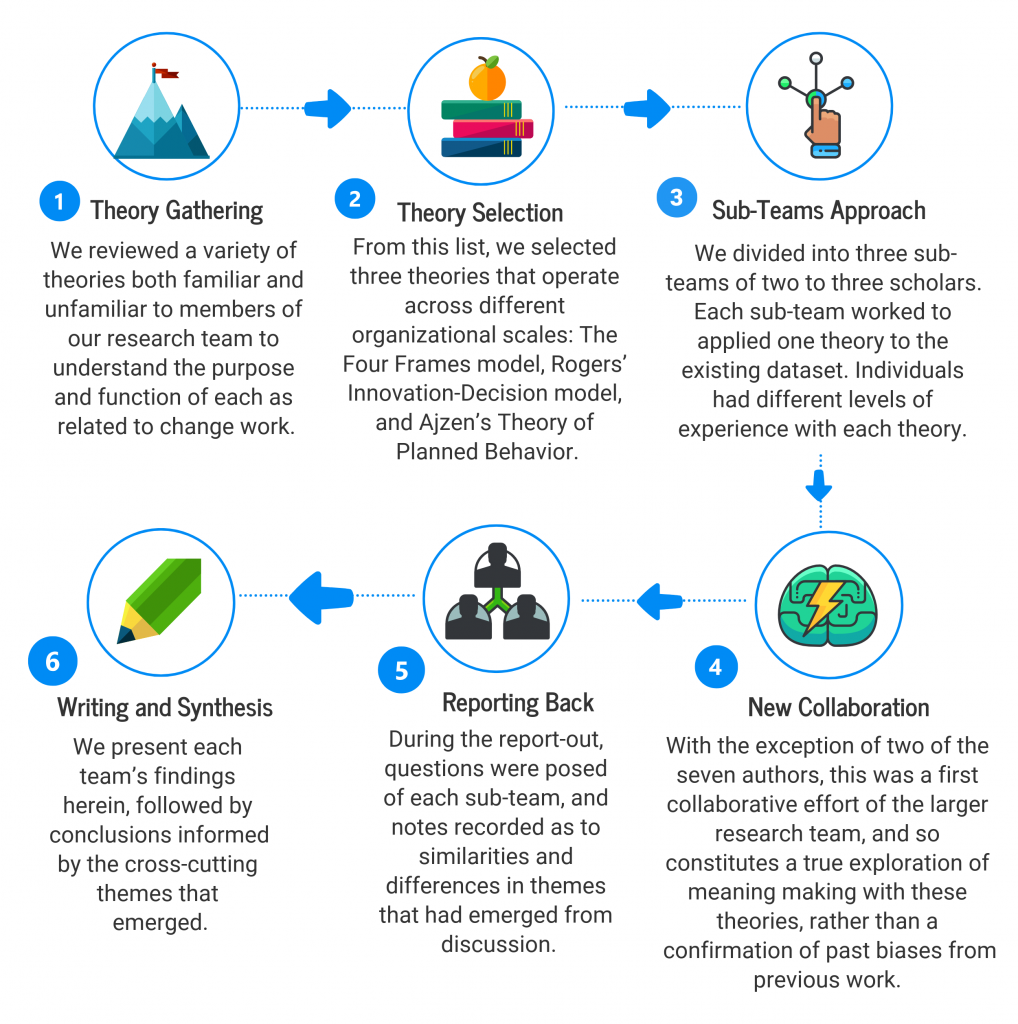
We divided into three teams of two to three scholars (see Figure 1). Each team applied one of the theories (section 2.2) to the data. Team members coded individually and then met to review applied codes, discussing challenges and emerging themes. This iterative cycle of analysis (moving back and forth between individual and team discussions) occurred multiple times so that each team co-constructed a common understanding of the data. Following analysis, each team then reported back to the larger group to provide a summary of the team’s findings.
We present each team’s findings below, followed by assertions informed by the cross-cutting themes that emerged.
3 Results and Insights
3.1 The Four-Frame Model
We applied Bolman and Deal’s Four-Frame model (2008) as adapted by Reinholz and Apkarian (2018) for higher education. This perspective of the Four-Frame model provides four perspectives from which a change initiative within a STEM department can be examined, namely people, structures, power, and symbols (Reinholz & Apkarian, 2018). These four frames constitute the culture in a department, which Reinholz and Apkarian define as “a historical and evolving set of structures and symbols and the resulting power relationships between people” (p. 3). We first attempted to apply the theory as a rudimentary codebook to characterize the barriers and drivers for change as identified by individual stakeholders within a department. This approach was not fruitful for two reasons. First, the descriptions of barriers and drivers in this dataset were brief, so it was difficult to know at which level they were operating (i.e., individual barrier vs. department or institutional barrier). Second, most of the barriers and drivers could be viewed through multiple frames. Ultimately, we shifted our approach to instead query the data to understand each response as evidence that an individual was viewing the change initiative through each of the four frames.
This approach yielded two new insights. First, individuals were more likely to attend to issues as they relate to structures and people, and less likely to attend to issues of power and symbols. For example, common responses included comments about who taught courses and potential collaborations, as well as curriculum concerns. Statements such as “More sharing of approaches & perspectives in common areas of teaching” and “Pressure to cover many topics” highlight this. Less common responses, such as “No current culture for this” and “Administrators with no real understanding of discipline specific issues could create an environment of rigidly applied but counter-productive standards” capture a focus on culture and positional power as drivers or barriers of change. This suggests changes to structures and people may be perceived as more accessible than shifts in symbols and power. Second, we surmised that as change agents, our work in responding to these barriers and drivers might begin by leveraging individuals’ perceptions of more “proximal” frames (i.e., structures and people) and work purposefully to cultivate thinking about how to view the change initiative through more “distal” frames (i.e., power and symbols). Our hypothesis is that individuals who can shift perspectives between all the frames will be more likely to support and sustain the change initiative. A limitation of the Four-Frame model is that it does not highlight readiness to change at the individual level. Change at the individual level is better understood through Roger’s (2003) Innovation-Decision model or Ajzen’s TPB (1991).
3.2 Rogers’ Innovation-Decision Model
Rogers’ (2003) Innovation-Decision model has been applied in diverse contexts to understand how individuals adopt an innovation. In the field of education, such an innovation could be new curriculum, classroom technology, or pedagogical strategies. The Innovation-Decision model identifies stages through which individuals progress as they decide whether or not to adopt an innovation: knowledge, persuasion, decision, implementation, and confirmation. However, an individual’s adoption of an innovation may be influenced by “prior conditions” such as previous experience with the proposed innovation, recognition of the need for innovation, level of innovativeness, or norms of the social system in which the innovation will occur (Rogers, 2003, p. 163). The model has subsequently been modified to explain the actions/decisions of faculty in higher education (Andrews & Lemons, 2017) and has been integrated with other models like Hall and Hord’s Concerns theory (1987) to identify specific apprehensions or concerns an individual or group may have regarding adoption of an innovation.
Hall and Hord (1987) identify concerns having to do with the self (need for additional information or a concern about persisting in the field), task (implementation of innovation), or impact (long-term effects of innovation). Similar to other researchers (e.g., McLean, 2005), we found it useful to pair Hall and Hord’s Concerns theory with Innovation-Decision because we could further categorize participants’ responses by type of concern (self, task, or impact). For example, the response, “Not so beneficial to me, personally, in that teaching is not in my experience a strong criterion for obtaining tenure and promotion” is aligned with Rogers’ prior conditions about the norms of the social system. While we are limited to the assumption that this respondent is not yet at the implementation stage, we can classify this statement with greater certainty as a self concern in which the respondent is addressing a concern about persisting in the field.
The attitudes expressed in the participants’ responses also provided insight into how a change agent might effectively address the barriers or concerns. In the previous example, we can surmise that a change agent might seek to create incentives for teaching effectiveness to motivate participant buy-in to institutional change efforts. In a second example, we can deduce that the respondent who indicates “Everyone has different ideas about teaching so why say one way is better than others” is in an early stage of the Innovation-Decision model (e.g., knowledge) and may benefit from an introduction to the literature on EBIPs. A limitation of both Rogers’ model and Hall and Hord’s theory was the finding that barriers and concerns align more clearly than drivers and concerns, thus making the model easier to apply to the barriers half of the dataset.
3.3 Ajzen’s Theory of Planned Behavior
While Roger’s Innovation-Decision model considers the individual level, the TPB is an interaction between the individual and the system in which the individual resides. According to the TPB, the individual’s intent to engage in a behavior is reinforced through continual feedback loops that connect their personal attitudes (based on behavioral beliefs and evaluations of outcomes), perceived norms (based on normative beliefs and motivation to comply), and perceived behavioral controls (based on control beliefs and perceived power) (see Figure 2). Note that the barriers and drivers do not hold equal weight for the individual and may influence one another.
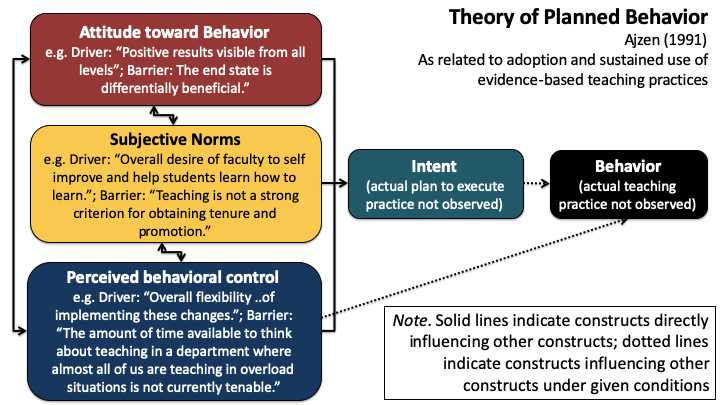
As with the application of other theories, the application of TPB to this particular dataset was difficult. However, we found it more challenging to code the barriers data than the drivers half of the dataset. Part of this challenge may come from the nature of the TPB, which examines the things (attitudes, norms, perceived behavioral controls) that determine behavioral intent. It is possible that respondents are inherently more likely to respond with positive beliefs about these constructs in the context of the study. Another possible explanation is that there are sufficiently high drivers in these two samples to positively influence behavioral intent, and that barriers would have been easier to code for a department more prone to negative beliefs about EBIPs. This could be further examined by documenting behaviors, or sampling of additional departments.
We tended to code barrier data primarily as perceived behavioral control. This included themes of time, class size, and personal motivation. The tenure/promotion process was frequently cited as something that seemed to touch on both behavioral controls and subjective norms (i.e., departmental expectations). If the individual perceived that they had less control of these things, it may be that they are less likely to intend and/or engage in the use of EBIPs. However, a noted limitation to examining these data alone is the absence of a second piece of data to confirm if these behaviors are actually occurring.
Most drivers we coded were consistent with attitudes (feelings; desires for change) or norms (expectations from others; adherence to those expectations). Within these codes, we noted that most drivers were attitude-based, not normative. This could be because teaching norms can be intangible and challenging to elicit (Fishbein & Ajzen, 2010). Conner and Armitage (1998) also note that norms may be the weakest of the TPB constructs in its ability to predict intent and behavior. Alternatively, the original question could have limited how faculty articulated norms; or, because the data we coded were all from one of two departments, faculty may have been influenced by similar norms, and therefore we had difficulty noting those themes in the dataset. If norms are intangible but present (i.e., implicit), we also wonder to what extent that faculty, subscribing to a norm of autonomy, have difficulty acknowledging how they are influenced by others (Hamilton, 2007).
One limitation in using TPB with this particular dataset is that the behavior itself is not captured. Likewise, it is unclear exactly whether participants intended to do the behavior of interest. A necessary next step for change agents is to understand whether the behavior itself was actually enacted, and whether any one lever or combination of levers was most central to driving or preventing change in this department. Though most barriers fell into the Perceived Behavioral Control category, it is possible that sufficiently positive drivers in the attitudes or norms categories helped to overcome those barriers. A potential intervention to explore would be to see how changes in attitudes can help faculty navigate barriers, perhaps through the normative influence of change agents. In essence, these leaders could support a personal norm of resilience to explicitly identify barriers and foster positive attitudes among other change agents in a department, so that others may adhere to those attitudes as well. For example, an intervention to help faculty re-conceptualize or expand upon their perceived behavioral controls around time, space, and tenure may be helpful. Although time is a common perceived barrier, it was not clear across all cases whether the time was a limitation for planning or enacting EBIPs. By helping faculty build supportive attitudes (e.g. moral obligation to teach with best practice; willingness to make mistakes in trying active learning), they can build a willingness to face constraints and achieve change in the classroom.
4 Conclusion
In well-designed research, theory is used to frame and design studies, guide data collection, and inform data interpretation. It is not surprising, then, that our post-hoc analyses with new theories presented challenges—with some theories easier to apply to the data (or half of it) than others. The raw data were collected to enumerate the drivers and barriers for a change initiative as perceived by individual faculty members. Yet the brevity of the individual responses meant that the contextual detail necessary to interpret the data from some theoretical perspectives was lacking and limited the degree to which new inferences might be drawn from the data. This held true even for theories that function to explain individual behavior (e.g., innovation-decision). In practice, change agents might similarly find that data collected to evaluate an initiative are insufficient. Using theory to select or design appropriate evaluation instruments can guard against this, especially if those instruments are initially field-tested to see if they are producing sufficient data. Our approach was instructive in thinking about how theory should be used to gather evidence of the efficacy of change efforts. We found that our use of Appreciative Inquiry throughout the process revealed several other lessons, which we describe below.
First, this process provided our research group with a broader sense of affordances and limitations of different theories. The scale of this project does not allow us to propose a definitive process for choice of theory for any given project. However, the appreciative inquiry provided each of us with insights that will inform our future use of theory. These include: On what level does the theory operate?, What kind of data would be needed to use this theory?, and What role does context play?
Second, the analysis conducted with each theory yielded concrete and valuable ideas regarding actions change agents might take, additional data to be collected, and/or new lines of inquiry for scholars. This suggests that an application of different theories may be useful for moving a study or implementation project forward, especially if it were conducted at a juncture when an expansion of ideas could help scholars or change agents move beyond a bounded view based on a single theory.
Third, the application of theories that operate at different levels of analysis revealed interesting ideas about what is important for change. No theory captures all the components of the complex system of higher education at once. However, using multiple theories provided a glimpse into different components of the system. For example, our analysis yielded insights about both individual perspectives and how those individuals viewed the larger context in which change was to take place. By exploring the dataset with the constructs of each theory, we gained insight into the whole. Further, the absence of depth in the dataset indicates that there is more to be uncovered and affirms what Bangera et al. discuss in their chapter of this volume, in that change is not linear and is more of a “squiggle.” Capturing the nuances that impact a change project is important in understanding how change occurs and may be sustained.
In the future, such explorations may yield insights into how to integrate different theories across different levels of the system. This invites scholars to move beyond expertise in a particular theoretical area because stretching ourselves to explore different theories gives us new perspective. The work presented here suggests that STEM education change scholars and agents should be familiar with a variety of theories. This would allow one to look for opportunities in which a particular result might be able to be examined through a different theoretical lens. As an example, the theory of change outlined in the Ngai et al. chapter of this volume provides indicators for a department’s readiness for change, which, if grounded in local context as recommended by Earl et al. (this volume), may better position a change effort for success. Such an activity could yield insights that may cross levels of focus, which may be particularly important as we seek to illuminate how change works across components of the larger system.
Our appreciative inquiry surfaced several important findings with respect to the use of theory in the analysis of an educational change project. Certainly our work reinforced that change scholars and agents should choose theories that operate with a focus aligned with one’s research question or intended scope of change. We argue, however, that change scholars and agents should also give attention to context and determine how much of the context needs to be considered for their work.
In summary, our exploration shows that the application of new theory provides a fresh perspective from which initial interpretations can be further inspected, alternative hypotheses generated, and new directions for change agents identified. We encourage other scholars to build on this work to illuminate additional insights of relevance to scholars and change agents seeking a richer understanding of the use of theory for STEM education change.
5 About the Authors
Mary E. Pilgrim is an Assistant Professor in the Department of Mathematics and Statistics and Associate Director of the Math and Stats Learning Center at San Diego State University.
Kelly McDonald is a Professor of Biological Sciences and the Director of the Science and Math Success Center for the College of Natural Sciences and Mathematics at California State University, Sacramento.
Erika G. Offerdahl is an Associate Professor of Biochemistry and Associate Director of Undergraduate Programs in the School of Molecular Biosciences at Washington State University.
Katherine Ryker is an Assistant Professor of Geoscience Education in the School of the Earth, Ocean and Environment at the University of South Carolina.
Susan E. Shadle is the Vice Provost for Undergraduate Studies and a Professor of Chemistry at Boise State University.
Amelia Stone-Johnstone is a Doctoral Candidate in the Math and Science Education Doctoral program at San Diego State University.
Emily M. Walter is an Assistant Professor of Biology and Director of the STEM Education Center at California State University – Fresno.
6 References
Ajzen, I. (1985). From intentions to action: A theory of planned behavior. In J. Kuhl & J. Beckman (Eds.), Action control: From cognitions to behaviors (pp. 11–39). Springer.
Ajzen, I. (1991). The theory of planned behavior. Organizational Behavior and Human Decision Processes, 50(2), 179–211.
Andrews, T.C. & Lemons, P. P. (2015). It’s personal: Biology instructors prioritize personal evidence over empirical evidence in teaching decisions. CBE-Life Sciences Education, 14(1), 1–18. https://doi.org/10.1187/cbe.14-05-0084
Austin, A. E. (2011). Promoting evidence-based change in undergraduate science education. National Academies National Research Council Board on Science Education. https://sites.nationalacademies.org/cs/groups/dbassesite/documents/webpage/dbasse_072578.pdf
Bangera, G., Vermilyea, C., Reese, M., & Shaver, I. (this volume). On the RISE: A case study of institutional transformation using idea flow as a change theory. In K. White, A. Beach, N. Finkelstein, C. Henderson, S. Simkins, L. Slakey, M. Stains, G. Weaver, & L. Whitehead (Eds.), Transforming Institutions: Accelerating Systemic Change in Higher Education (ch. 6). Pressbooks.
Bolman, L. G., & Deal, T. E. (2008). Reframing organizations: Artistry, choice, and leadership. Jossey-Bass.
Conner, M., & Armitage, C. J. (1998). Extending the theory of planned behavior: A review and avenues for further research. Journal of Applied Social Psychology, 28(15), 1429–1464. https://doi.org/10.1111/j.1559-1816.1998.tb01685.x
Cooperrider, D., & Srivastva, S. (2017). The gift of new eyes: Personal reflections after 30 years of Appreciative Inquiry in organizational life. In Research in organizational change and development (Vol. 25, pp. 81–142). Emerald Publishing Limited. https://doi.org/10.1108/S0897-301620170000025003
Cooperrider, D., & Srivastva, S. (1987). Appreciative Inquiry in organizational life. In W. Pasmore & R. Woodman (Eds.), Research in organization change and development (Vol. 1, pp. 129–169). JAI Press
Dormant, D. (2011). The chocolate model of change. lulu.com.
Earl, B., Viskupic, K., Marker, A., Moll, A., Roark, T., Landrum, R. E., & Shadle, S. (this volume). Driving change: Using the CACAO framework in an institutional change project. In K. White, A. Beach, N. Finkelstein, C. Henderson, S. Simkins, L. Slakey, M. Stains, G. Weaver, & L. Whitehead (Eds.), Transforming Institutions: Accelerating Systemic Change in Higher Education (ch. 1). Pressbooks.
Fishbein, M., & Ajzen, I. (2010). Predicting and changing behavior: The reasoned action approach. Routledge. https://doi.org/10.4324/9780203838020
Hall, G. E. & Hord, S. M. (1987). Change in schools. State University of New York Press.
Hamilton, N. W. (2007). Faculty Autonomy and Obligation. Academe, 93(1), 36–42.
Henderson, C., Beach, A., & Finkelstein, N. (2011). Facilitating change in undergraduate STEM instructional practices: An analytic review of the literature. Journal of Research in Science Teaching, 48(8), 952–984. https://doi.org/10.1002/tea.20439
Howard Hughes Medical Institute (2019). Summer Institutes on Scientific Teaching. https://www.summerinstitutes.org/
Kezar, A. (2011). What is the best way to achieve broader reach of improved practices in higher education? Innovative Higher Education, 36(4), 235–247. https://doi.org/10.1007/s10755-011-9174-z
McLean, J. (2005). Addressing faculty concerns about distance learning. Online Journal of Distance Learning Administration, 8(4), 1–13. https://www.academia.edu/download/48971937/mclean84.pdf
Ngai, C., Corbo, J. C., Quan, G. M., Falkenberg, K., Geanious, C., Pawlak, A., Pilgrim, M. E., Reinholz, D. L., Smith, C., & Wise, S. (this volume). Developing the Departmental Action Team theory of change. In K. White, A. Beach, N. Finkelstein, C. Henderson, S. Simkins, L. Slakey, M. Stains, G. Weaver, & L. Whitehead (Eds.), Transforming Institutions: Accelerating Systemic Change in Higher Education (ch. 5). Pressbooks.
Reinholz, D. L., & Apkarian, N. (2018). Four frames for systemic change in STEM departments. International journal of STEM education, 5(1), 1–10. https://doi.org/10.1186/s40594-018-0103-x
Reinholz, D. L., Pilgrim, M. E., Corbo, J. C., & Finkelstein, N. (2019). Transforming undergraduate education from the middle out with Departmental Action Teams. Change: The Magazine of Higher Learning, 51(5), 64–70. https://doi.org/10.1080/0091383.2019.1652078
Rogers, E. M. (2003). Diffusion of innovations (5th ed.). The Free Press.
Shadle, S. E., Marker, A., & Earl, B. (2017). Faculty drivers and barriers: Laying the groundwork for undergraduate STEM education reform in academic departments. International Journal of STEM Education, 4(1), 8. https://doi.org/10.1186/s40594-017-0062-7